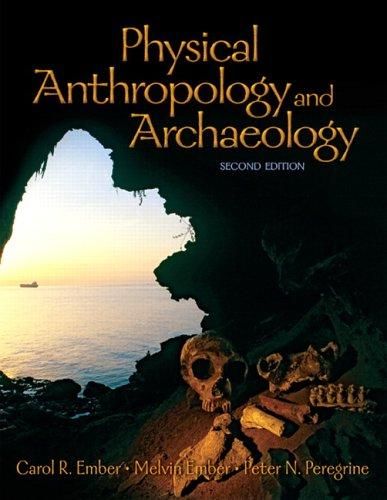
two programs, two examples. The first one is
going to be structure. And in the slides material you
saw yesterday was information on the website that
you can go to, to download the structure
package and program. And what we have here on
the computer is that file.
And once you have it, if you look in the
contents there is this icon that has the various
colored bars typical of structure output. But you can run structure and this is the page
that will come up. You can go under file and
examples of all of these are in that manual tutorial
whatever you wanna call it that you also had. Here, you can go to new project,
click on that and it's going to ask you for information.
You can name the project, what I would suggest you
do is use your initials and call it test something
any arbitrary name. I used Test 2 because I've
also run a Test 1 before. You need to tell the program
where information is the program and its necessary components
are in the desktop in this case, in the structure folder,
and you select that. The data file, we have
loaded in here a data file.
We will browse also
on the desktop and in the structure folder we
have a folder called "data." And because this data set
is related to the examples in the FROG database that
we'll talk about later. We call it FROG 1943
and that is entered. Next, you want to enter
the number of individuals. Well, we called it FROG 1943, because that's the
number of individuals.
Everybody is a deployed two
chromosomes these are all the symbol. The number of loci is 39. And what we have used is minus 9 to represent a missing
data value, not everybody will have
results for every locus. At this step 3, we
simply click and note that we have put the marker
names into the data file.
And here we've also put
in the individual ID. We put in the putative
population of origin. It's in fact not putative
because we know where all of these individuals came from. And we're going to
click the flag that will use that for display.
The calculations in structure
do not use population origin but we can display
by population. We can look at the data format
and it tells us how many lines of data, how many
columns, et cetera. We finish and here is a
summary of what we've put in, our project name, the
pathway, the data source, the number of individuals
and the nature of the data. And we can proceed and here
is what we see, the data.
We have all of the marker names,
we've got the population ID. And the flag for ordering them. Now, at this point, we
need to set the parameters. And there are no
parameters already stored, it's a new project.
We have no parameters. Now, the parameters are going to tell us how long
to run the program. This works on Markov
chain Monte Carlo which means it chooses
values in the parameter space to gather a sense of where the
parameters are best during a burning period. And then it follows that up
with a match finer sampling around the better values.
So, because I know this
computer runs reasonably fast, I'm going to put in 4,000 and
4,000 for a total of 8,000. In most runs for really
getting a better idea where speed is indeed
important 10,000 burn-ins, 20,000 MCMC repetitions is sort
of the minimum one word used but is usually quite sufficient. So we want to name
this parameter set, I'm going to give it the same
name as the project but that's by no means necessary,
it's an arbitrary name so that you can come back
to this parameter set. So now, we're really
ready to run the program, depending upon your computer
for reasons I do not understand.
Sometimes you can immediately
start to run the project, other times you actually have
to save at this point, get out, come back in and
restart the project. So I'm going to do that, we're
going to save the project and then we're going to exit and then we're just
going to come back in. And we are back here
and now at file instead of a new project we're going to
open a project and we're going to the desktop, we're going
into structure and we're going to look at Test 2 and here
is an SPJ that's the project, structure project. And we start it, there
is our data set again.
And now, we can go in
and try to start a job. And it's going to ask us, what are parameters
are, and the K values. The K values tell you how many
different clusters you want to fit these individuals into. And structure will
then find the best way to fit these individuals,
given that it's on Monte Carlo approximation.
So every maybe a
little bit different. So, we know that these data are
reasonably good at clusters of 6 or 7 so we can try both of them. And the number of iteration
because it say local maximum, it'll be different
potentially at every single run. So normally, one would do 10
or 20 runs and find the one with the highest likelihood as the examples are
in that tutorial.
>> But here, we're just
going to do 2 replica. So we're talking now about
a total of 2 replicas at K equals 6, 2 root K equals
7, and we're going to start. And you can see in the lower
left it's actually started, it's already done 200, 300
of these 4,000 burn-in runs. So we simply have
to wait a minute.
We're going to do 8,000,
4,000 burn-in 4,000. So we're already 1/8 of
the way through there and you may want to--
we're almost at 4,000, the burn-in has completed. Now, we're beginning the
finer runs, and here you see in the right hand column is the
estimated log of the likelihood of the particular parameters
you see in that line of F1 through F6, and they're
all hovering somewhere around minus 51, 300. So the first run has
completed and it's now started on the second run at K equal 6.
But, while that's running,
we can go up here and look at the results of the first run. There is a lot of
text here with a lot of different bits
of information. I'm just going to scroll down
to this point fairly close to the front where the mean
value of the log likelihood which is a value we're
going to want to use to compare different runs. We want the run that has
the highest likelihood.
And if you saw they are
fluctuating around minus 51 300 and here is the exact result
for these set of data. But, what's more interesting
is to just look at it. And if we go up to the bar plot and show it depending upon the
sides of screen you've got, you maybe able to extend
this out to the right. We don't have room for that.
So we'll have to
scroll through it. This is individuals each
little vertical line and you can see some
of them here as thin distinct vertical lines
is a different individual. And they are colored by which of the six clusters
they best fit into. Here there in their input
order, but we're now going to group them by
those population ID's.
We just put in numbers, so you
have to use the number code page that you-- you have in the
folder where we give the number and what population
it corresponds to. Here, we've got a
whole bunch of blue. Let's quickly scroll across, we're going to have six
colors corresponding to the different populations,
a different clusters that we're trying to group
these individuals into. You see some populations
are only one color, others are a mess.
But let's look at
what those are. The first, the blues
are the Africans, 1 are the Biaka Pygmies, 7
are the Yoruba from Nigeria, 12 are the Hausa, another
Nigerian peoples group that does not speak
a Bantu language. And they are slightly
distinct, doesn't show up with these markers. If you look at the
bottom of the screen, you see the second
run has finished and it's rapidly writing
out all of the results and then we'll start
the third run.
In the meantime, we can
look at this first run, 13 and 14 are the Maasai
from Northern Tanzania, and the Chagga from the
based of Mount Kilimanjaro. They are the porters for
those who hike up Kilimanjaro. And 16 are the Sandawe
a click-speaking, hunter-gatherer group
also in Tanzania, 17 are African-Americans. And suddenly you can begin
to see individual differences that can be fairly significant.
And we know African-Americans
are admixed. We will talk more
about that later. Here, 20 are the Ethiopians. If you remember,
or go back and look at the tree structure I
showed, this population, these are the same individuals
same population are intermediate between sub-Saharan
Africa and Southwest Asia.
And here most people are closed
to 50/50, that may be admixture but its also equally
likely beforehand to be that these individuals are-- that this whole population
is intermediate and structure has had
lots of individuals in Southwest Asia
here on the right, and lot's of sub-Saharan
Africans on the left, and it doesn't have
enough degrees of freedom or enough clusters
to allocate these to their own cluster it's
simply places them in between. Whereas in African Americans
we know there is admixture, and these may very well on an individual basis represent
the amount of admixture. One can't say arbitrarily. What is admixture and
what is intermediate? So 25 and 26 are the Samaritans
and the Yemenite Jews, 28 are the Druse and then
31 are the Ashkenazi Jews.
And they have a lot of green
and a lot of yellow and a lot of individual variation
but clearly also have a lot of Southwest Asian
signature if you will. How much again this is admixture on an individual basis
is difficult to say but it's not surprising that
there is European gene flow into Ashkenazi Jews and
yet as a population. They clearly maintain a signal
if you will of Southwest Asia, 39 are the Adygei a Southern
Southeastern European population, just at the North
of the Caucasus mountains on the shores of the black sea,
the east shore of the black sea, it's the only Caucasian
population we have. You heard me say
how much I think of Caucasian is a racist term in geographic sense,
these are Caucasian.
42, Are Hungarians
and as we move through this Europeans we see
the Irish have a lot of yellow, we have still got a little
bit of this pink showing up the European-Americans
are fairly "admixed" and then there is a
little more green as we get into the northeastern
populations if we look here at 49 and 50 those
are Finns and Danes. 51 Are the most northeastern
population that Komi that exist on both sides of
the Euro mountains across the northern
edge of the Euros. 56 Are Khanty a side a western
Siberian population that falls in between any of
the clusters we have. 57 Are the Keralites from
South India and they have a lot of these pink showing similarity
to the southwest Asian's, Middle East and not
to Northern Europe.
Then, we get into East Asians
and like blue the pacific 103, 105 are not distinguished here and we then have the 4
Native American populations that are quite distinct. So let's take a quick look now
at other runs the second run at K equal 6, we can look and
see if it's a different pattern. A lot of them are
different patterns. Here, it looks pretty similar,
we end up with the same colors, here we're getting
into northern Europe and quite complex the
Khanty and the Keralites, let me move this up, and
then we get into East Asia and the Native Americans.
So here we got two patterns
that were really quite similar but very different
patterns can occur. If we stop here and
now go to K equals 7, one of them has already
completed and the other is getting
close to completion. So now we're allowing
one more cluster. And here we see all
of the Africans, there are still roughly half
and half happen the Ethiopians.
Now, the Samaritans are
very clear and things begin to degrade meaning cluster
cannot allocate these individuals into a
single group very much. Now, in part of Europe, we're
getting three colors red, pink, actually the light
blue and an orange. What does the orange represent? The orange seems to be representing far
northwestern Europe the Irish. The whole job just completed so
we can look at the second run at K equals 7 in a moment.
And here the Komi are not orange but 50 are the Danes are
very much like the Irish. Again, this is complex
East Asia maybe a hint that the pacific are a
little different but not much and then the new world. We can look at the fourth run. Here, we see very much the same
thing we we're seeing we're getting 3 different
colors in Europe, the same sort of pattern.
The colors are different because
each time you run structure it arbitrarily chooses
a set of colors. There are ancillary
programs disrupt by Rosenberg will allow one to make all the colors
roughly correspond when the clusters are the same. And here we get essentially the
identical pattern we had before. So unfortunately, in
this run I'm not able to show how different
the patterns can be.
But they can be very different and we can email
you this data set and you can play
with it if you want. And the data will clearly in some cases give
very different results. At the end of the tutorial
package is the example of eleven different runs
of a superset of this data with a few more individuals
in it that will allow one
together sense of the different likelihood's and the different
patterns that can occur. Now, structure is
not a good method for assigning an
individual to a population, you'd have to put your
unknown into a large data set with a good number
of markers and see which cluster the person fell
out in because in that long list of information that comes with
the cluster run, you can scroll down and here you can
see for each unique ID.
Of an individual you
have the probabilities or the relative clustering
in each of the 7 clusters. And so, clearly here
are several individuals from the same population and
they mostly cluster in cluster-- well, 1, 2, 3, 4 cluster 4
of 7, that's population 26 so these are the Yemenite Jews. But, some of them don't,
here's one that falls primarily in cluster 1 not cluster 4. So instead of using
cluster to analyze data, we find it's very useful for
trying to identify the sets of markers that are
most powerful in subdividing populations.
This is a set selected to be
able to make some differences across Europe as well as
more continental groups and indeed it does that. What is better for trying
to look at the ancestry of a given individual is to
use a livelihood approach with relative likelihood's and
that's what we have attempted to implement in a very early
stage of our FROG database. So let me connect to the
internet, bring up FROG. And then we can start on the
second part of this exercise.
[ Music ].
Tidak ada komentar:
Posting Komentar